AI: The elephant in your lab!!
Dr Ashok Kumar, President-CRD, IPCALaboratories explores the impact of AI in scientific research and drug development, from predicting protein structures to self-driving chemistry labs. He points out that AI is revolutionising how we approach complex tasks, and in future,AI-driven innovations may reshape our understanding of science and enhance productivity
Technologies are evolving quickly, and some of them could significantly alter the kinds of goods and services we are accustomed to as they are so disruptive. Disruptive technologies, whatever their nature, have greatly improved our work and lifestyle. Computers, the Internet, Google search engines, and smartphones, while partially to blame for the demise of many indispensables in the recent past, like the gramophone, telegraph, and typewriter, have had a profound impact on both our professional and personal lives.
The most significant shift that is currently occurring is the result of artificial intelligence (AI) and machine learning (ML), which have made thinking machines more dependable and efficient than people at handling tasks like gathering and aligning data, analysing it, and making better decisions. Like humans, it gains efficiency over time by constantly learning from experience, but, on the contrary, without incurring errors (positive), in whatever activity it undertakes.
Two of the most significant turning points in the history of artificial intelligence were Deep Blue’s 1997 victory over chess champion Garry Kasparov and Deep Mind’s 2016 victory over Go champion Lee Sedol, both of whom were thought to be beyond the capabilities of the most advanced computer systems. The key characteristic of the Alpha Go algorithm is that, instead of only reacting to the information that is provided, it learns the game’s rules, uses tactics that have never been considered by humans, and even finds new rules as it goes.
Another example of a coming technology that will undoubtedly alter the direction of the automotive industry and those connected to it is the development of AI-powered self-driving cars.
Nonetheless, Chat GPT, which debuted at the end of 2022, might be regarded as a true disruption of the present. In just five days following its release, over a million people looked at it for different reasons. It genuinely deserves the acclaim it has received, as it not only provides concise and straightforward answers to the questions but also explains the ideas in plain language. Not only does it create original ideas, but it also offers advice on how to plan events and businesses, composes articles, essays, and poems on the subjects assigned, solves challenging arithmetic problems, and even helps select appropriate gifts for specific occasions.
Launched on March 14, 2023, ChatGPT-4 is its next avatar and is 40 per cent more effective than its predecessor at providing factual responses. It is trained in around 26 languages, has a larger general knowledge base consisting of approximately 100 trillion parameters compared to 175 billion, and can solve complex problems. It is not just eighty percent more intelligent at spotting unnecessary queries, and it can also take image inputs in addition to text. In contrast, GPT-3.5 is limited to processing text inputs. Because ChatGPT-4 is interactive, it answers follow-up inquiries, acknowledges errors, refutes false premises, and grows from its mistakes.
When compared to the caliber of human professionals, GPT-4 has scored in the 90th percentile on US bar exams and shown above-average undergraduate ability in medical assessment exams and coding, which calls for strong math and science knowledge.
It is easy to see from these advancements that AI/ML will continue to advance in capabilities, and depending on how we interpret these developments, their future iterations will continue to astound or surprise us.
AI and scientific research
Traditionally, people have thought that creative and innovative vocations, including the fine arts, science, and complicated decision-making requiring critical thinking, are exclusively human domains and will never be impacted by AI. But it doesn’t seem like this theory is correct, as exemplified by a few recent developments in chemistry and related fields.
New drug discovery: Finding new targets is essential to developing new drugs, but doing so demands a difficult-to-get structural understanding of proteins and how they behave. In this regard, the DeepMind algorithm AlphaFold has significantly enhanced the ability to predict the three-dimensional (3D) structures of proteins from their amino acid sequences. It disclosed the projected structures of over 200 million proteins that are currently known to science or humanity in July 2022. It’s crucial to remember that, thanks to this new technology, determining a protein’s 3D structure may now be completed in a matter of hours as opposed to years.
For example, when protein structure is unknown, AlphaFold can be used in conjunction with two other AI tools, PandOmics and Chemistry 42, to enable an end-toend procedure for the new discovery. Target discovery engine PandOmics sheds light on the association between a target and a disease, while Chemistry 42 proposes binding sites for putative drug candidates by analyzing AlphaFold’s predicted protein structure (ChemWorld ’40, March ’23). Because AlphaFold2 can anticipate multiple protein configurations, it is not only helpful for researching protein dynamics but also has the potential to revolutionise drug development by identifying new targets.
New drug development: The field of new drug development is expanding its use of AI and big data in a synergistic way. This includes lead optimisation and the prediction of the pharmacokinetics (ADMET) of possible drug candidates, in addition to early drug discovery that focuses on creating new targets or hits. Clinical trial patient subgroup selection and trial prediction are now commonly done by AI, which helps significantly cut down on costs, time, and, of course, late-stage failures. It is crucial to understand that bringing a new medication to market can cost up to several billion US dollars and take 12 to 15 years. According to the CPhI Annual Report of 2023, which is based on data from 250 international pharmaceutical companies, more than half of all approved medications would use artificial intelligence (AI) in their development or production within ten years.
Because AI can process and analyse large, complex data sets to find patterns that are invisible to the human eye but essential for accurately determining the underlying cause of an illness and, of course, choosing the best course of treatment, it has already proven to be a game changer in the healthcare industry.
Enzyme function prediction: CLEAN, a novel deep learning system, makes predictions about the function of enzymes by analysing their amino acid sequences. This online tool, which is free to use, can assist in swiftly characterising and identifying the appropriate enzyme for the intended production of a substance or chemical. (www.science.org/doi/10.1126/science.adf2465).
Enzyme engineering: An enzyme created by an AI/ML system that breaks down plastic may depolymerise a whole plastic tray in less than 48 hours. It accomplishes this for fifty-one (51) distinct PET products more quickly and even at lower temperatures than the next best thing that was created by humans (Nature 604, 662, 2022).
De Novo enzyme design: The development of unique proteins with novel behaviors, functions, or goals has been made possible by directed evolution. However, the lack of knowledge of the physicochemical forces at work necessary to give a protein its threedimensional shape continued to hinder the design of a protein from scratch. With the aid of recently developed AI technologies, this now seems to be feasible as well.
For instance, researchers can construct novel protocols utilising various algorithms, such as the open-source AI platforms ColabFold, and ColabDesign to produce structures, design sequences, and fold to validate proposed sequences that could create the designed proteins. It is crucial to understand that researchers can now employ these methods without being constrained by their understanding of the physics and chemistry of these molecules. A new generative AI model called CHROMA has recently been shown to create artificially intelligent proteins with programmable features that have the potential to be curative (C+EN, Nov. 2023, 24).
Synthetic route design: Synthia, Chemetica’s newest incarnation, recommends synthetic routes for complicated molecules using a retro-synthetic methodology. It is based on 100,000 hand-coded rules and a database. Additionally, novel strategies that are almost unheard of in the literature are suggested by this Merck KGA tool.
ROBOT RXN, the algorithm developed by IBM, is comparable to Synthia, makes > 4 million reaction predictions, and is already used by > 30000 chemists worldwide.
Reaction condition predictor: The AI empowered robot that learns from the outcomes of the reaction not only predicts the best conditions but also carries out the experiments using the predicted conditions to give double the human optimised yield in Suzuki- Miyamura coupling reactions.
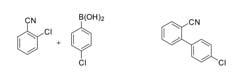
Furthermore, Chemists can improve reaction quality and prevent certain side reactions by using the cheat sheet that was developed from meta-analyses of all the literature on Buchwald-Hartwig (>62000) reactions. In order to promote the intended changes more successfully, the algorithm also anticipates the use of suitable reagents and conditions.
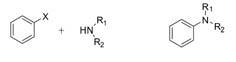
Another machine learning system called Labmate makes recommendations for the temperature, solvent concentration, and anticipated duration of a given reaction in order to help optimise the reaction conditions and produce the highest yields. Additionally, it suggests doing experiments to try to improve upon the current state of affairs (DOI: 10.1016/j.xerp.2020.100274).
Enantioselectivity prediction: Trained to determine the activation energy for the competing R and S pathways, which can then be translated to enantiomeric excess (ee), is quite remarkable in predicting the enantioselectivity of an asymmetric transformation (DOI:10.1038/s 41560-021- 00813-w).
Atom tracking: Without human assistance, an ML algorithm called RXN Mapper can track atoms in the product from reactants with >99% accuracy (Nature Communications (DOI: 10.1038/41467-019- 09990-2). Catalyst discovery: In its first project, the robot chemist in Andy Cooper’s lab at the University of Liverpool carried out 688 experiments in eight days by modifying ten variables, ultimately finding a photo-catalyst mixture that was six times more active than the original formulations (Chem World P23, Feb. 2023).
Stereospecific C-C bond formation: The core component of the naturally occurring neurotoxic substance Kalkitoxin, which is produced by a cyanobacterium, is made using a fully automated robotic platform that enables carbon chains to be grown one atom at a time with control over chain length and stereochemistry.
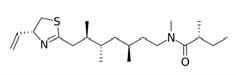
Self-driving chemistry labs
An end-to-end AI research assistant called Coscientist, which decides reaction conditions and also writes codes for automated systems based on single English Prompt has recently demonstrated Suzuki and Sonogashira coupling reactions without any human intervention. Interestingly, it designed experiments suitable enough to be performed within the hardware that was available, executed them, and analyzed the results, as expected from a chemist to give the output (Nature 2023, 62Pg, 570; Chem.World P41, Feb. 2024). Another advanced LLM version of Chatbots, namely ChemCrow, has also demonstrated capabilities similar to or better than those of Coscientist. When asked to make an insect repellant, ChemCrow first carried out a literature search to find one, translated its chemical name into SMILES, designed the synthesis, and operated the robotic laboratory system at IBM to produce a physical sample of DEET, a known insect repellent, without any human assistance (DOI:org/10.48550 /avXiv.230 4.05376).
Yet another example of a self-driving lab is AlphaFlow, which produces derived Quantum dots by managing more than 40 variables of the reaction condition, allowing it to discover > 1012 potential sequences in a month, and that too using a novel recipe (C+EN P4, April 17/24, 2023). Like the previously mentioned cases, it completed the task without the need for human assistance.
The final word
There are enough reasons in the aforementioned description to conclude that AI has already made deep inroads in areas of scientific research. It is not difficult to imagine that artificial intelligence (AI) will revolutionise scientific research by bringing new insights from experiments because of its ability to learn more than a billion times faster, gobble up unlimited data, retrieve information at the speed of light, and draw quick conclusions from massive and even disorganised datasheets that are incomprehensible to the human mind. I-agent systems, which are quickly approaching, will soon provide the current LLMs with abilities like self-thinking, brainstorming, and refining data while handling or researching complex tasks! This is just the beginning. Imagine what AI will be able to do when a highly developed generative AI architecture backed by a quantum computer endowed with all the principles, rules, and knowledge of the chemical or biological sciences becomes accessible!
Be assured, this will occur sooner than anticipated!
One might think that AI will never surpass human intelligence or be able to completely replace people (hope it proves true in the future), one thing is certain: those who understand when and how to use AI to get the most out of life and work will undoubtedly replace those who haven’t learned to make friends with this behemoth!!